- 1Institute for Biophysical Dynamics and the James Franck Institute, The University of Chicago, Chicago, IL, United States
- 2Molecular and Cellular Biology Department and John A. Paulson School of Engineering and Applied Sciences, Harvard University, Cambridge, MA, United States
- 3Institute of Science and Technology Austria, Klosterneuburg, Austria
- 4Department of Biological Sciences, Columbia University, New York, NY, United States
- 5Instituto de Ciencias Físicas and Centro de Ciencias de la Complejidad, Universidad Nacional Autónoma de México, Cuernavaca, Mexico
In Gram negative bacteria, the multiple antibiotic resistance or mar operon, is known to control the expression of multi-drug efflux genes that protect bacteria from a wide range of drugs. As many different chemical compounds can induce this operon, identifying the parameters that govern the dynamics of its induction is crucial to better characterize the processes of tolerance and resistance. Most experiments have assumed that the properties of the mar transcriptional network can be inferred from population measurements. However, measurements from an asynchronous population of cells can mask underlying phenotypic variations of single cells. We monitored the activity of the mar promoter in single Escherichia coli cells in linear micro-colonies and established that the response to a steady level of inducer was most heterogeneous within individual colonies for an intermediate value of inducer. Specifically, sub-lineages defined by contiguous daughter-cells exhibited similar promoter activity, whereas activity was greatly variable between different sub-lineages. Specific sub-trees of uniform promoter activity persisted over several generations. Statistical analyses of the lineages suggest that the presence of these sub-trees is the signature of an inducible memory of the promoter state that is transmitted from mother to daughter cells. This single-cell study reveals that the degree of epigenetic inheritance changes as a function of inducer concentration, suggesting that phenotypic inheritance may be an inducible phenotype.
Introduction
Advances in time-lapse microscopy (Locke and Elowitz, 2009) and substrate-printing (Bennett and Hasty, 2009), combined with new fluorescent reporter proteins, have facilitated the characterization of stochastic phenotypic behaviors (Cluzel et al., 2000; Elowitz and Leibler, 2000; Le et al., 2005; Le et al., 2006; Guet et al., 2008; Chang et al., 2022) that are often masked by population measurements (Balaban et al., 2004). Most of these methods depend upon measuring exclusively the concentration of fluorescent reporters. The accumulation and dilution of these reporters obscure time-dependent fluctuations at short timescales. These fluctuations are less of a limitation at long timescales in studies of bistable systems such as the ara and lac operons (Novick and Weiner, 1957; Siegele and Hu, 1997; Vilar et al., 2003) or bacterial persistence (Balaban et al., 2004), in which positive feedback loops lead to clearly distinct “on” and “off” states (i.e., digital networks). However, most gene regulatory networks produce a continuously varying distribution of outputs (i.e., analog networks), and thus detecting the transmission of information from cell to cell especially at cell division can be very difficult (Austin et al., 2006; Taniguchi et al., 2010). In order to detect inheritance in networks with analog outputs, we have developed a technique using promoter activity time-series in single-cell lineages to quantify epigenetic inheritance for a gene regulatory network (Figures 1A–D). We use the promoter of the multi-antibiotic resistance (mar) operon from Escherichia coli fused to a gene coding for a fluorescent protein to test our technique. The study of single cell lineages reveals the presence of an inducible inheritance phenotype in the expression of the mar promoter as measured from a very low-copy reporter plasmid.
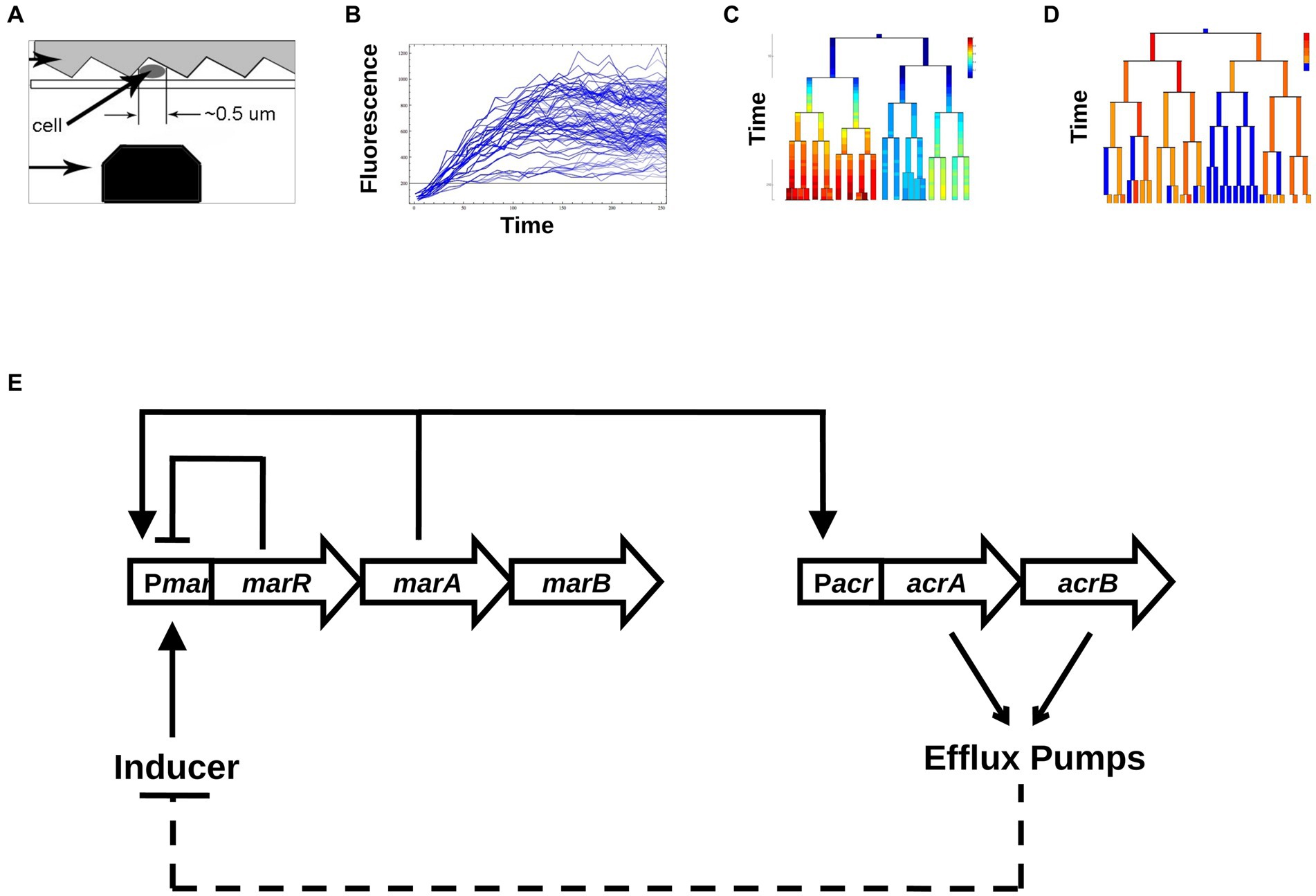
Figure 1. Schematic map of our experimental and analysis approach. (A) Data collection. Single Escherichia coli cells are trapped between a patterned agarose pad and a cover slip, and are excited via a blue laser that moves along the length of the cell using a scanning nano-positioning stage. (B) Conversion into time series of total fluorescence. From the raw data, time series of fluorescence are produced across growing colonies. (C) Changes in [YFP] as a function of time across generations of growing and dividing bacteria. (D) Binarization of promoter activities, into active and inactive cells. (E) The core transcriptional network of the mar system includes the repressor MarR that regulates the marRAB operon. In the presence of the inducer salicylate, MarR repression is abolished, allowing MarA to activate its own expression and the expression of the acrAB operon. The acrAB genes form the efflux pump complex AcrAB-TolC. Efflux pumps create a negative feedback on marRAB activity due to efflux of the inducer salicylate.
The mar operon also denoted as marRAB, consists of three genes: marR—a repressor, marA—an activator and marB—whose function is not fully understood. The biology of the mar operon has been extensively studied at the population level (Martin et al., 1996), yet little is known about the dynamical aspects of this inducible gene regulatory network at the single-cell level. Through the direct and indirect action of MarA on about 60 different genes, mar represents one of the largest regulons of E. coli (Barbosa and Pomposiello, 2005). One of the main targets of MarA is activation of the expression of the multi-drug resistance efflux pump operon acrAB (Li and Nikaido, 2004). In the absence of an inducer, the mar network is characterized by a negative feedback loop produced by MarR self-repressing the mar operon (Alekshun and Levy, 1997; Figure 1E). In the presence of an inducer, such as salicylate, MarR repression is relieved, and the activator MarA initiates its own expression, constituting a positive feedback loop. MarA also activates the acrAB and tolC operons, that together encode the AcrAB-TolC efflux pumps (Martin et al., 2008), the main multi-drug resistance determinant of Gram negative bacteria. Over time, the accumulation of efflux pumps reduces the intracellular concentration of inducer, which constitutes a negative feedback loop by renewing the repression of the mar operon by MarR. Thus, the activation of the mar system confers multi-drug resistance (George and Levy, 1983) that is eliminated when the pumps are no longer expressed (Alekshun and Levy, 1997; Le et al., 2006). The reversible nature of this resistance suggests that individual cells may have widely ranging responses to a steady input (Lewis, 2007). Interestingly, the alternating behavior of the positive and negative feedback loops (Figure 1E) has the potential to reverse the activation of the mar promoter even under a steady level of inducer (Thomas and Richelle, 1988; Le et al., 2006; Aracena, 2008).
Here, we measured activity of the mar promoter in single cells using a confocal scanning volume across E. coli colonies growing as linear micro-colonies, starting from a single “mother” cell. The mar promoter controls the expression of a yfp-venus reporter with very fast maturation time (Balleza et al., 2018) expressed from a very low-copy plasmid with SC101* ori (Lutz and Bujard, 1997). Using the promoter activity reported via YFP expression, we constructed genealogy trees describing promoter induction for individual lineages of E. coli. We used an algorithmic process to binarize the output of the promoter and then calculated a measure for the degree of epigenetic inheritance of each individual lineage at various inducer concentrations. Surprisingly, we found that the coefficient of variation (CV) of expression depended in a non-monotonic way on inducer concentration. Based on our analysis, we found that the degree of epigenetic inheritance changed as a function of inducer concentration, suggesting that phenotypic inheritance may be an inducible phenotype within an analog-output gene regulatory network.
Materials and methods
Strains and plasmids
We used the E. coli K-12 strain Frag1B (Le et al., 2005) for all experiments. Plasmid pZS*1mar-venus: We replaced the gfp from pZS*1R-gfp (Guet et al., 2002) with the venus-yfp gene using KpnI and HindIII sites. We PCR amplified the mar promoter, containing the marbox and the two MarR operators, from the Frag1B chromosome. Since one operator for MarR overlaps with the marR coding region, we retained the first seven amino acids at the N-terminus of MarR and fused them to Venus-YFP (Martin and Rosner, 2004). We mutated the wild-type GTG start codon of marR to ATG by changing G with A in the primer. We cloned the mar promoter into the XhoI and KpnI sites of pZS*1R-venus. The KpnI site introduced a Gly-Thr linker between MarR and Venus-YFP. The pZS*1mar-venus plasmid contains AmpR and has a SC101* ori that maintains the copy number at 3–4 copies/cell (Lutz and Bujard, 1997). We transformed all strains by electroporation.
Growth conditions
We grew cells overnight at 30°C in Luria-Bertani broth (LB) containing 100 μg/mL ampicillin (Sigma Aldrich, St. Louis, MO, United States). We diluted the overnight cultures 1:600 in fresh LB media and harvested cells after an additional 3.5 h at 30°C. The optical density (OD) of the final cultures varied between 0.12–0.15 as measured at 600 nm.
Sample preparation for microscopy and submicron-groove fabrication
We prepared fresh 3% low melting agarose (Fisher Scientific, Pittsburgh, PA, United States) in LB in a 70°C water bath. We mixed salicylate from a 1 M sodium salicylate (Fluka, Switzerland) stock solution with 100 μL LB and added the mixture to 5 mL LB-agarose and swirled vigorously for 1 min. in order to achieve rapid and homogeneous mixing. We poured 100 μL of this 3% agarose-LB as a 1 cm2 gel slab onto an optical grating (300 lines/mm, blaze angle 26°, Bausch and Lomb, Rochester, NY, United States). The gel pad solidified for 20 min in the dark at room temperature, imprinting the grooves of the optical grating in the gel. We placed 0.3 μL of freshly harvested E. coli cells on top of a thin coverslip (Fisher Scientific, United States) sealed with wax to an aluminum holder. We placed the agarose-LB slab on top of the 0.3 μL droplet of cells. Due to surface tension, most cells aligned themselves in the submicron-grooves. The sample was sealed and immediately placed on the heating stage of an inverted Olympus X71 microscope (Olympus, Japan). We began collecting data within 5–10 min of the sample set up.
Number of cells measured
For each salicylate inducer condition we measured 10 different linear micro-colonies. Experiments were carried out over different days. For 0 mM we measured 392 mother-daughter pairs, for 0.25 mM 430 pairs, for 1 mM 510 pairs, for 3 mM 457 pairs.
Data collection and processing
To measure fluorescence from individual cells, we focused blue laser light (Sapphire 488 nm, 20 mW, Coherent, Santa Clara, CA, United States) into a diffraction-limited spot (width = 0.4 μm; Guet et al., 2008) and scanned cells at 4 μm/s using a nano-positioning stage (Physik Instrumente, Auburn, MA, United States). Emitted photons were collected in a confocal geometry and detected with an avalanche photodiode (ALV, Langen, Germany). This scanning procedure is minimally invasive and accurately measures fluorescence intensity within individual cells (Guet et al., 2008). We scanned each cell every 5–10 min. We aligned the scanning trajectory of the laser spot perfectly along the submicron-grooves using the nano-positioning stage. Fluorescence data was stored for further analysis.
Each scan produced a fluorescence profile of individual cells from a micro-colony. Fluorescent data was binned into 0.4 μm pixels. Because the excitation spot was small (width = 0.4 μm), cell cleavage sites appeared as sharp dips in the fluorescence profile while cell bodies appeared as long, bright fluorescence plateaus. Cell length was the distance between two consecutive cleavage sites. The difference in length between two consecutive measurements was used to estimate the cell growth rate. To produce genealogy trees, we used a visual interface (in house software, Matlab). Using this program, we manually picked cleavage sites from the intensity profiles produced by the scanning and assigned and tracked cell lineages. The intracellular concentration of fluorescent proteins was given by the brightest pixel. The fluorescence intensity was directly related to the intracellular concentration of fluorescent molecules. The promoter activity was the change in fluorescence intensity between two time points corrected for by a dilution factor that accounts for growth. We assigned each cell a single value for promoter activity between divisions.
Data analysis
Binarization
We binarized promoter activity as either ON (active) or OFF (inactive) using a threshold. Activity was ON if the cell had promoter activity at least two standard deviations above the mean of the distribution of activities of un-induced cells. Activity was OFF if the promoter activity was below this threshold.
Domain size and degree of inheritance
For each tree, we defined a sub-tree as two or more cells that were directly connected (through mother-daughter or sister–sister relationships) and all shared the same promoter activity, i.e., ON or OFF. For each tree, we computed the mean sub-tree size of ON and OFF sub-trees, <ON > and < OFF>, respectively. The mean sub-tree size is a measure of how large the average sub-tree is in which a randomly selected cell finds itself on a genealogy tree. It is defined as:
where is the number of ON domains that contain s cells, and represents the definition of a mean domain size in percolation theory (Stauffer and Aharony, 1994). For each tree we calculated the product <ON > <OFF>. For a given inducer concentration, the degree of inheritance was defined as the average domain size product, or divided by the number of trees, N.
Computational model
We implemented a discrete-time branching process in which all cells in the population divide simultaneously. Each cell can be in one of two possible states: active (on) or inactive (off). When a cell divides, each of its two daughter cells can be active or inactive depending on the state of the mother cell. To implement the model, four conditional probabilities must be defined. Denoting as DC and MC the daughter and mother cells, respectively, the four conditional probabilities are: , , , and . Only two of these four probabilities are independent since, , and . Therefore, the complete branching process is thoroughly characterized by the two probabilities and . These two probabilities were computed from experimental data for different inducer concentrations. Once the relevant probabilities are fixed, the branching process starts with one cell in the “on” state assuming that this cell has been placed in a medium with nonzero inducer concentration. From there, the branching process evolves.
Results
Measuring marRAB promoter induction in individual bacteria across linear micro-colonies
We cloned the full length mar promoter upstream of Venus yfp (Nagai et al., 2002), on a very low copy plasmid with SC101* origin (3–4 copies per cell; Lutz and Bujard, 1997).
The SC101* origin has a very tight regulation of partitioning. Consequently, partitioning governed by SC101* origin is accompanied by much lower plasmid noise than that of higher copy number plasmids (e.g., colE1 origin) that rely on unregulated random partitioning. The fast maturation of the Venus-YFP chromophore allows us to report (Balleza et al., 2018) changes in mar promoter activity within a few minutes. We monitored salicylate-induced expression of the mar promoter as a function of time in wild-type E. coli cells at the single cell-level across several inducer concentrations that cover the dynamic range of previous population measurements (Rosner, 1985; Cohen et al., 1993). To limit cell–cell contact, we restricted cell growth to one dimension by growing bacteria as linear colonies within long micro-grooves imprinted into agar. This geometry ensures that all bacterial cells are grown in similar micro-environmental conditions. To ensure the genetic and epigenetic homogeneity of a linear colony during growth, we restricted our measurements to micro-grooves that were initially occupied by only a single bacterium. By scanning the colonies with a focused blue laser and collecting the emitted fluorescence with a photodiode (Guet et al., 2008), we monitored the expression level of YFP in individual bacteria exposed to a steady level of the inducer salicylate ranging from 0 to 3 mM. We measured the concentration of the YFP reporter, [YFP], at 5–10 min intervals in individual cells across the linear micro-colonies. At the single-cell level, we observed that [YFP] varied several-fold within colonies, even between siblings (Figure 2A). Using the coefficient of variation (CV) as a quantitative estimate of the cell-to-cell variability, we found the highest variability in [YFP] at 1 mM salicylate (CV = 0.35 at 1 mM; CV ≤ 0.25 for the other inducer concentrations; Figure 2B). By contrast, the average [YFP] across all colonies reached a well-defined steady state for each concentration of inducer about 120 min after induction (Figure 2C).
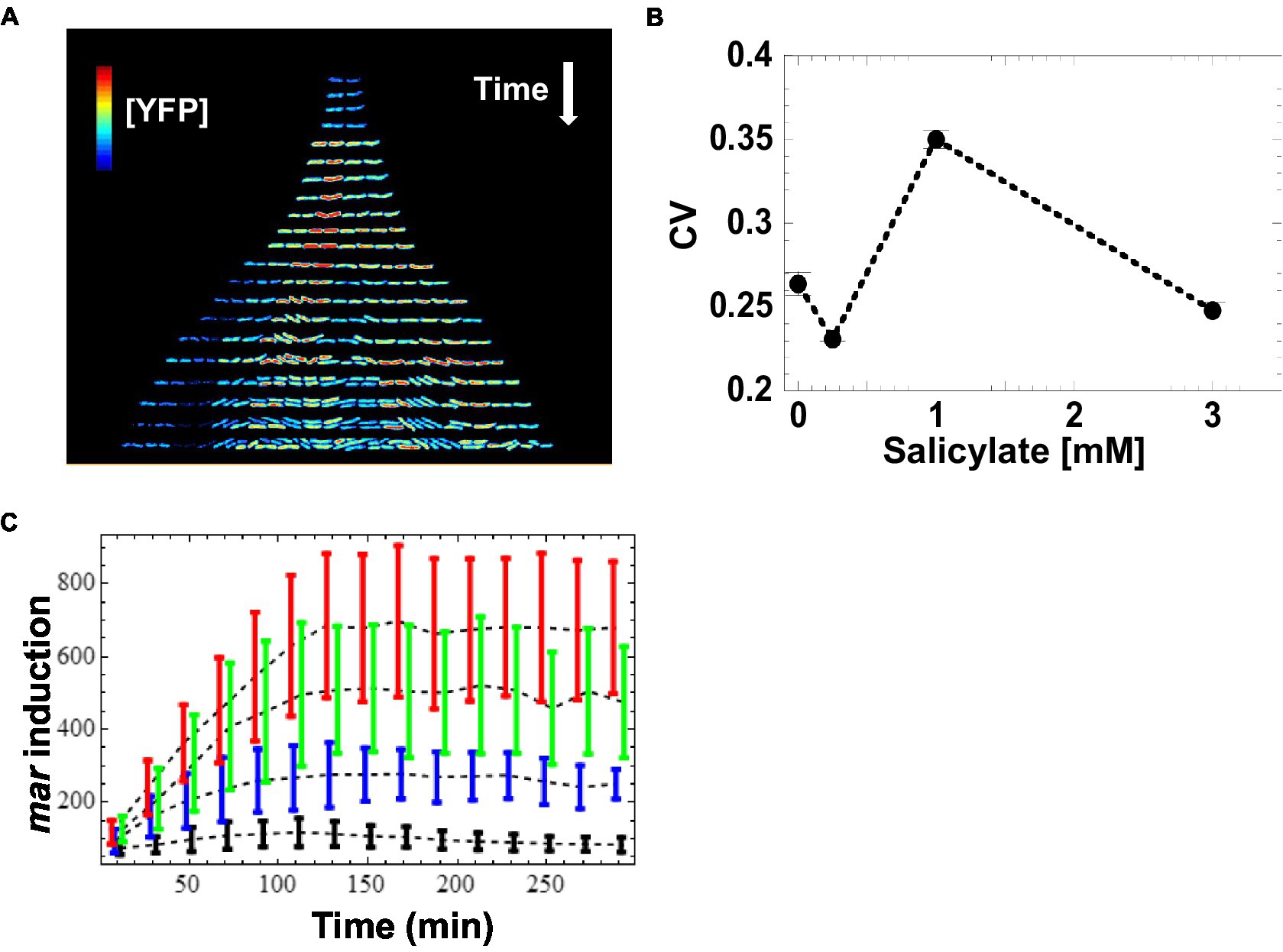
Figure 2. Induction of mar promoter with salicylate. (A) Induction of the mar promoter in individual cells of a linear colony. Single colony images are separated by ~20 min. The heat map shows fluorescence intensity (Red = High, Blue = Low) from YFP expression driven by the mar promoter activity in individual cells exposed to a steady level of salicylate (1 mM). Induction starts at t = 9 min. (B) Mean coefficient of variation of [YFP]. YFP fluorescence from single cells was averaged for 10-min intervals and the coefficient of variation across the interval was calculated. The mean coefficient of variation across the interval 120 to 300 min was then calculated. Error bars represent the standard error. (C) Induction kinetics of the mar promoter at the population level. YFP fluorescence from single cells was averaged for 10-min intervals, reporting mar promoter activity as a function of time. Four different salicylate concentrations were used: 0 mM (black), 0.25 mM (blue), 1 mM (green), 3 mM (red). Error-bars are the standard deviation from the distribution of single cell measurements.
Monitoring gene expression across generations
Given the increase in CV value for the intermediate inducer value (1 mM) we observed, we wanted to find out whether this variation was a result of random fluctuations in individual bacteria, or if this effect was due to a transmission of cytoplasmic information from a mother cell to its daughters. To characterize how mar promoter gene expression changed through cell lineages, we monitored [YFP] across generations within the linear colonies and we represent the changes in activity using genealogy trees (Figure 2A). We generated the trees by presenting the time series of [YFP] concentration for a single lineage under each inducer level, with the concentration of [YFP] denoted from low (blue) to high (red). Both, the absence of salicylate (0 mM) and high (3 mM) concentration of salicylate led to homogeneous [YFP] across several generations of bacteria grown from an initial mother cell (Figure 2A; Supplementary Figures S1A,G). Remarkably, an intermediate salicylate concentration (1 mM) resulted in heterogeneous distribution of [YFP], ranging from very high (as observed with 3 mM salicylate) to very low (as observed with no salicylate; Figure 2A; Supplementary Figure S1E). The observed heterogeneity in gene expression forms sub-trees with high and low levels of [YFP] that coexist within a micro-colony, which is suggestive of an inherited gene expression state and which helps explain the much higher CV levels observed in Figure 2B.
Binarization of promoter activity and quantification of inheritance
The [YFP] measurements do not give a direct measure of when the promoter is on or off, as the cells grow and thus accumulate and dilute YFP proteins (Figure 3A). We therefore estimated the mar promoter activity by monitoring temporal variations of [YFP] while accounting for changes in cell volume due to growth. To simplify our analysis, we used a threshold to binarize mar promoter activities as active or inactive within genealogy trees. In the non-induced condition, the mar promoter exhibited a natural leakiness (characteristic of most bacterial promoters) that produced a basal level of promoter activity. The threshold was chosen such as to be between one and two standard deviations away from the mean value of the non-induced state (0 mM salicylate) and one standard deviation away from the mean of the highly induced state (3 mM salicylate; Figure 3B). Thus, cells with promoter activities below the threshold had an inactive mar promoter, while those with activities above the threshold had an active mar promoter. This binarization allowed for simple counting of active cells and inactive cell sub-trees (Figure 3C).
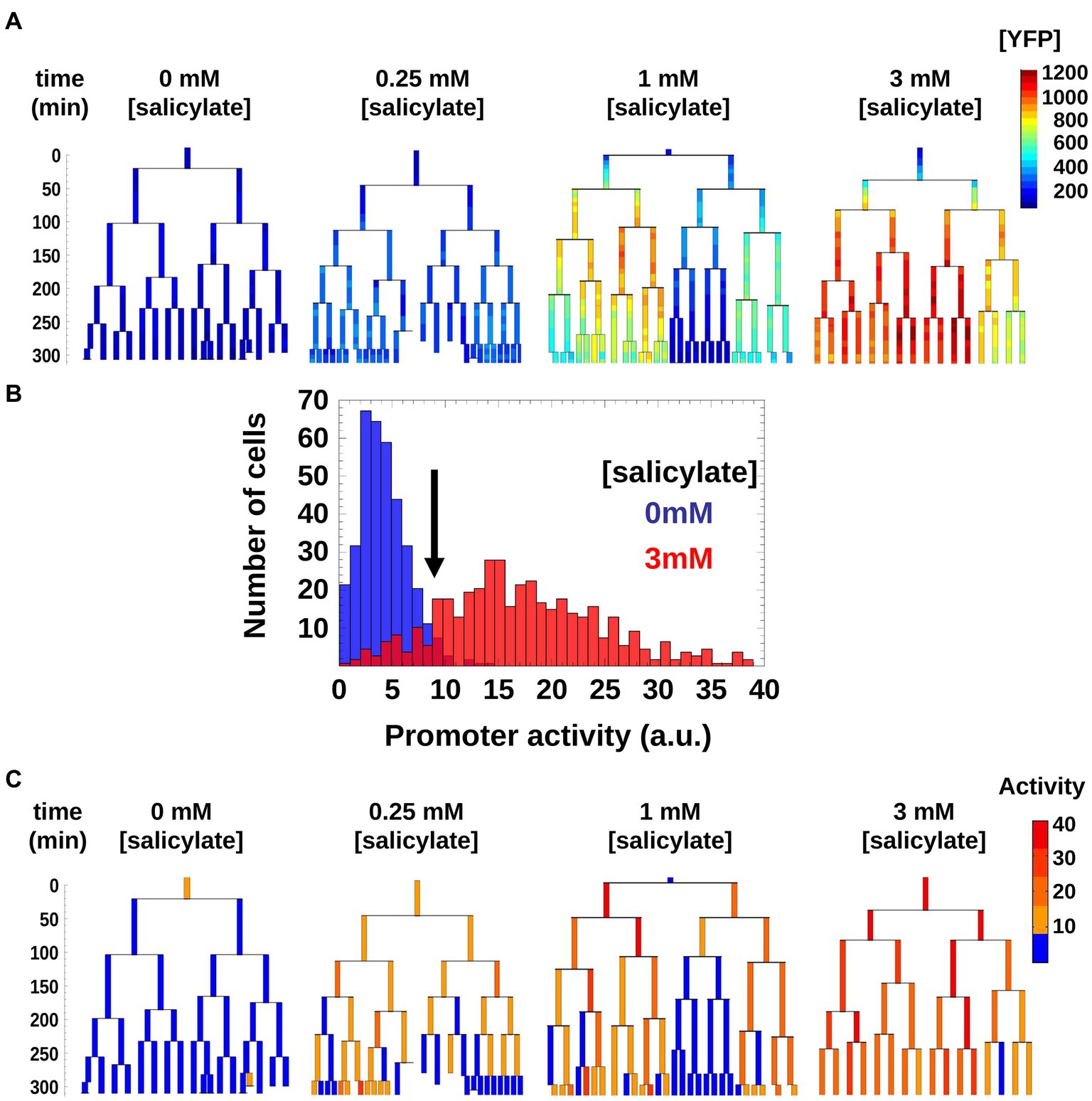
Figure 3. Genealogy trees of mar promoter induction in wild-type cells. (A) Single cells with a plasmid carrying yfp-venus regulated by the mar promoter were monitored through several generations in the presence of the inducer salicylate. The genealogy tree follows the induced level of YFP concentration through the generations of a colony. Constant salicylate exposure began at time t = 0. Heat-map scale uses arbitrary fluorescence units to show low (blue) to high (red) [YFP]. (B) Distribution of mar promoter activity across a population of non-induced 0 mM salicylate (blue) and fully induced 3 mM salicylate (red) cells. The promoter activity is estimated as the temporal derivative of the measured YFP concentration corrected for cell growth. The black arrow shows the threshold between active and inactive promoter states defined at two standard deviations from the mean of the non-induced cell distribution (blue). (C) Genealogy trees of mar promoter activity for different inducer concentrations. The vertical axis represents time in minutes. To binarize the promoter activity we used the threshold from (A). Below the threshold, the mar promoter is inactive (blue), above the threshold the promoter is active (yellow, orange, red).
As is apparent in Figure 3C, in the 1 mM condition, there is a coexistence of larger sub-trees of active and inactive cells, which form a patchy structure on the genealogy tree. In order to characterize this patchiness that is a signature of heterogeneity, we calculated the product of the average sizes of active and inactive sub-trees in a genealogy tree, and call this domain size (see Materials and methods). As the sub-trees are the result of an underlying inheritance process, the domain size serves as a measure of inheritance. If the average size of either active or inactive sub-trees is close to zero, then the degree of inheritance is small. When both active and inactive sub-trees are large, the degree of inheritance is high. We found that the observed inheritance was highest in genealogy trees with cells induced at intermediate levels (Figure 4A). This measure was insensitive to specific thresholds of promoter activity (Supplementary Figure S2).
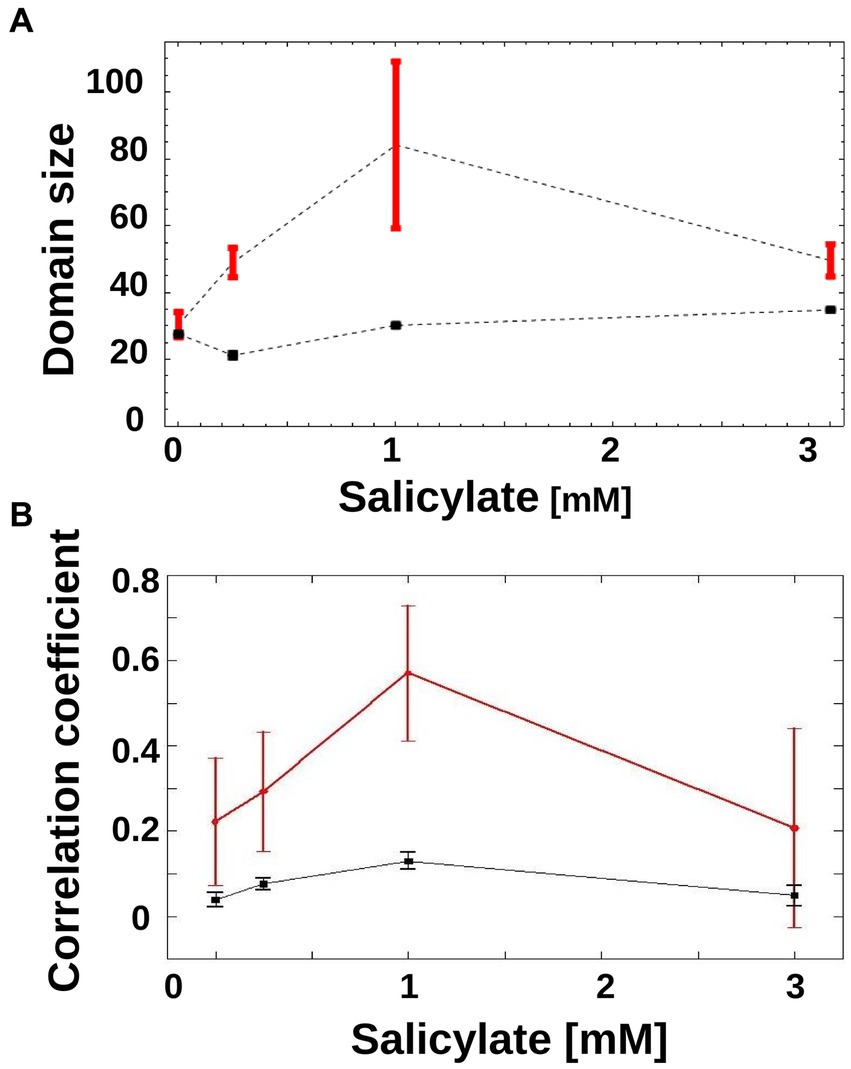
Figure 4. Inheritance of mar promoter activity. (A) Domain Size of trees is shown for wild-type genealogy trees (circles) and randomly reshuffled genealogy trees (squares) at different inducer concentrations. Error-bars are the standard error. (B) Correlations between mother/daughter promoter activity is shown for wild-type genealogy trees (red circles) and randomly reshuffled genealogy trees (black squares) as a function of increasing inducer. Correlation coefficients were calculated for mother/daughter pairs when expression has reached a steady state, in the interval of 120 to 250 min. N = 165 (number of mother/daughter pairs) for 0 mM salicylate, N = 180 for 0.25 mM salicylate, N = 90 for 1 mM salicylate, N = 72 for 3 mM salicylate. For reshuffled trees, the activities of each tree were shuffled within the tree itself, to generate 100 instances per tree using the same tree structure. Error-bars are 95% confidence intervals.
A different way of measuring inheritance of promoter activity from mother to daughter cells is to determine the correlation coefficient of the activity, which can also be done without the need to binarize the promoter activity. As can be seen in Figure 4B, the correlation coefficient between mother and daughter promoter activity displays a clear peak at 1 mM, and thus confirms the result obtained with the domain size measure.
Observed inheritance is not a result of random switching
If promoter activity switches randomly at each cell division, then the activity across trees would exhibit a “speckled” or “salt and pepper” appearance, and thus a noisy distribution. Alternatively, if cells inherit the ancestral promoter activity state, then trees would display large sub-trees of similar promoter activity. A detailed molecular model of mar operon induction would leave many parameters free because reaction rates and concentrations, which govern the dynamics of mar induction, have been only partially quantitatively characterized (Martin and Rosner, 1995).
To test if random switching alone can explain the observed promoter activity distribution, we generated randomized activity trees and calculated their domain size product. In order to render the theoretical result of the genealogy trees as relevant as possible to the experimentally obtained trees, we constructed each tree by randomly placing active and inactive cells on the leaves of the tree while keeping the number of active and inactive cells identical to those from the empirical genealogy trees. For all non-zero inducer concentrations, the randomized trees had a similar domain size product (Figure 4A, black squares) that was always lower than that of the experimental trees (Figure 4A, red circles). This pattern was robust to specific thresholds of promoter activity (Supplementary Figure S2).
Moreover, we asked if the induction patterns observed within genealogy trees could be captured by a simple toy model based on a Markovian stochastic process, wherein the size of the sub-trees is governed by cell switching from “on” to “off” and vice versa. For such a model, we hypothesized that at each generation, a cell has a given probability of switching the mar promoter activity of its mother from inactive to active (or from active to inactive). The switching probabilities depend on the inducer concentration and can be inferred directly from experiments (Figure 5A). Using the experimental switching probabilities for each inducer level as parameters for the toy model, we found that in agreement with the experimental trees (Figure 4A), the simulated trees exhibited the highest inheritance for intermediate inducer levels (Figure 5B).
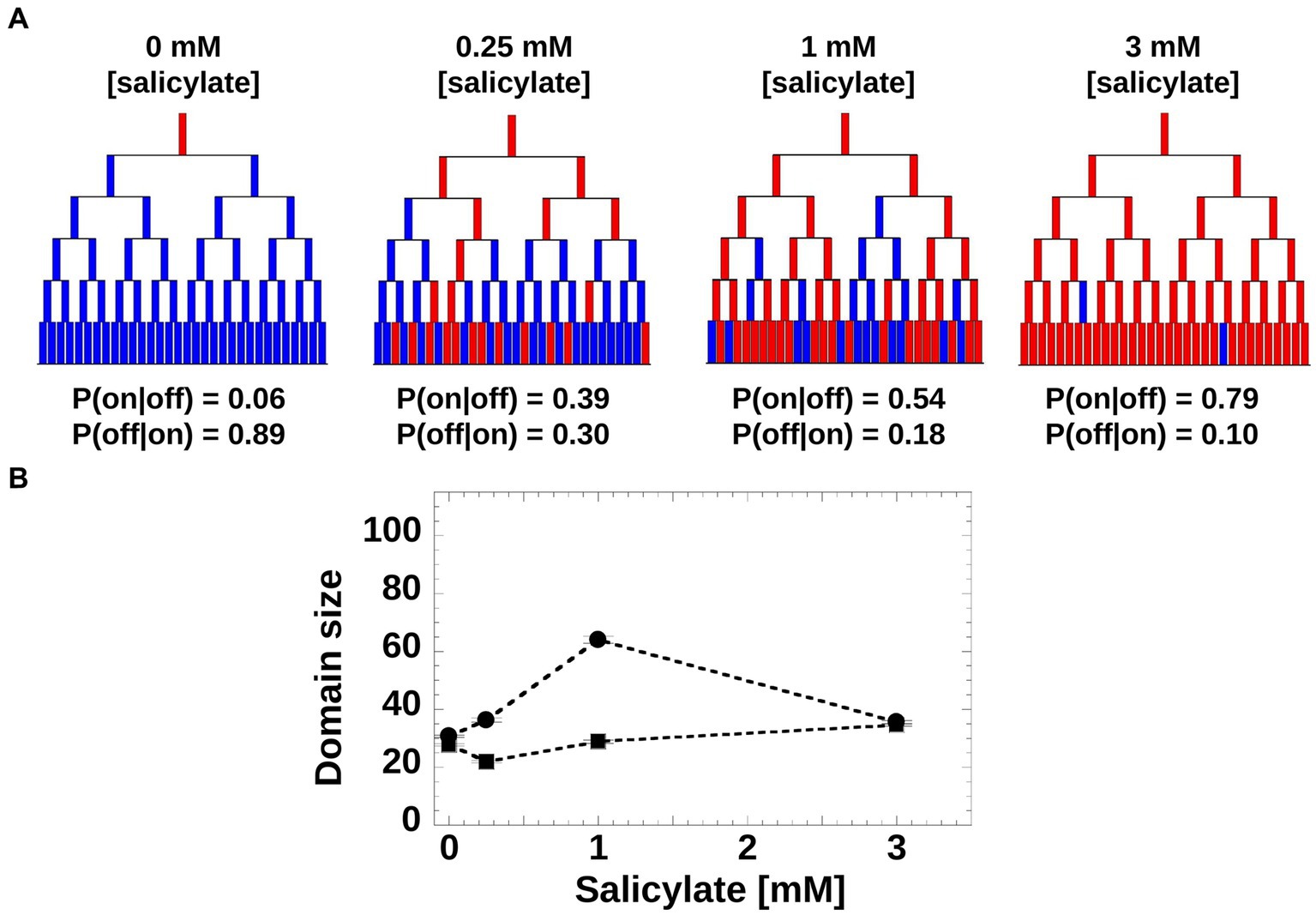
Figure 5. Toy model of mar promoter activity. (A) Toy model of mar operon induction. Cells can switch between active and non-active states with conditional transition probabilities P(on|off) and P(off|on). Values of the probabilities were determined from the experimental data. The genealogy trees follow cells through generations and illustrate switching between an inactive (blue) and active (red) promoter. (B) Domain Size product of trees was computed from 200 model simulations using the experimentally-derived conditional probabilities, P(on|off) and P(off|on; circles), and non-conditional probabilities, P(on) and P(off) when the inheritance strength is zero (squares).
Discussion
Our experimental technique has shown that phenotypic inheritance is also a measurable quantity in gene regulatory networks with analog output, and not only in bistable genetic networks. We have shown that inheritance may be sensitive to conditions, such as the induction level in the mar operon, which remains undetectable when measurements are performed at the population level (Cohen et al., 1993). The standard picture of the transcriptional regulation by MarR and MarA of the marRAB and acrAB operons has been established mostly through population level experiments (Martin et al., 1996). Based on these experiments we hypothesized that, under steady level of induction, the mar promoter from single cells would also exhibit a steady level of activity. Surprisingly, our experimental approach showed that even well after the promoter reaches its steady-state value measured at the population level (~120 min post induction), some single cells had an inactive mar promoter. Monitoring promoter activity through multiple generations revealed an unusual pattern of activity in induced cells within each isolated bacterial colony. We found that within lineages, cells with similar promoter activity form sub-trees across generations, suggesting an inducible inheritance where the activity of daughter cells reflects the promoter activity of the mother cell. Surprisingly, the level of inheritance peaks for an intermediate inducer concentration of 1 mM in E. coli cells.
This inducible inheritance is more subtle and transient than in all-or-none examples, such as the ara and lac operons and bacterial persistence and has therefore been more elusive and difficult to trace. Here, by measuring promoter activity across lineages and by simplifying the output by means of binarization, we were able to measure the degree of cell-to-cell inheritance. This technique should be broadly applicable, to a number of gene regulatory networks, using readily available plasmid reporter libraries (Zaslaver et al., 2006), e.g., flagellar assembly cascade (Kim et al., 2020), ribosomal assembly and metabolic networks. Using full lineages instead of solely the mother cell, could be applied at a high-throughput level using promoter libraries, wide-field fluorescence microscopy, and automated image-processing software. Using this type of systems approach could reveal heretofore unknown inheritance phenotypes in the gene regulatory networks of E. coli and other single-celled organisms.
While single-cell studies have recently have shown that the mar operon controlled efflux system AcrAB-TolC could be a source of heterogeneous phenotypes (Bergmiller et al., 2017; Meouche and Dunlop, 2018; Wen et al., 2018), the mechanistic origin of the inheritance we uncovered through our experimental and theoretical analysis remains an open question. For one, the intricate network of negative and positive feedbacks that the marRAB and acrAB operons form (Figure 1E) is by its very topological construction prone to complex dynamics behavior. What adds extra complexity to this already rich dynamics, is the recently discovered biased partitioning of the AcrAB-TolC pumps at old poles (Bergmiller et al., 2017), which in itself is also an inheritance effect. Thus, maybe what we observe here could be a superposition of two types of inheritance mechanisms and quantitatively unraveling the contribution of each will be an experimentally and theoretically challenging task. Lastly, that salicylate induces inheritance effects in the activity of the mar operon should pique the interest of clinicians who assess the effects of aspirin on the mar operon induction in microbiome bacterial residents.
Data availability statement
The raw data supporting the conclusions of this article will be made available by the authors, without undue reservation.
Author contributions
CCG and PC designed the research. CCG, LB, and PO performed the research. CCG, LB, PO, MA, and PC analyzed the data. CCG, LB, PC and PO wrote the paper. All authors contributed to the article and approved the submitted version.
Funding
This work was supported by NIH P50 award P50GM081892-02 to the University of Chicago, a catalyst grant from the Chicago Biomedical Consortium with support from The Searle Funds at The Chicago Community Trust to PC, and a Yen Fellowship to CCG. MA was partially supported by PAPIIT-UNAM grant IN-11322.
Acknowledgments
We thank Raluca Vescan for her kind help with image processing software. We also thank Wendy Grus and Kathleen Dave for editorial assistance.
Conflict of interest
The authors declare that the research was conducted in the absence of any commercial or financial relationships that could be construed as a potential conflict of interest.
Publisher’s note
All claims expressed in this article are solely those of the authors and do not necessarily represent those of their affiliated organizations, or those of the publisher, the editors and the reviewers. Any product that may be evaluated in this article, or claim that may be made by its manufacturer, is not guaranteed or endorsed by the publisher.
Supplementary material
The Supplementary material for this article can be found online at: https://www.frontiersin.org/articles/10.3389/fmicb.2023.1049255/full#supplementary-material
References
Alekshun, M. N., and Levy, S. B. (1997). Regulation of chromosomally mediated multiple antibiotic resistance: the mar regulon. Antimicrob. Agents Chemother. 41, 2067–2075. doi: 10.1128/AAC.41.10.2067
Aracena, J. (2008). Maximum number of fixed points in regulatory boolean networks. Bull. Math. Biol. 70, 1398–1409. doi: 10.1007/s11538-008-9304-7
Austin, D. W., Allen, M. S., McCollum, J. M., Dar, R. D., Wilgus, J. R., Sayler, G. S., et al. (2006). Gene network shaping of inherent noise spectra. Nature 439, 608–611. doi: 10.1038/nature04194
Balaban, N. Q., Merrin, J., Chait, R., Kowalik, L., and Leibler, S. (2004). Bacterial persistence as a phenotypic switch. Science 305, 1622–1625. doi: 10.1126/science.1099390
Balleza, E., Kim, J. M., and Cluzel, P. (2018). Systemtic characterization of maturation time of fluorescent proteins in living cells. Nat. Methods 15, 47–51. doi: 10.1038/nmeth.4509
Barbosa, T. M., and Pomposiello, P. J. (2005). “The mar regulon,” in Frontiers in antimicrobial resistance: a tribute to Stuart B. Levy, eds D. G. White, M. N. Alekshun and P. F. McDermott (Washington, DC: ASM Press).
Bennett, M. R., and Hasty, J. (2009). Modelling microfluidic devices for measuring gene network dynamics in single cells. Nat. Rev. Genet. 10, 628–638. doi: 10.1038/nrg2625
Bergmiller, T., Andersson, A. M. C., Tomasek, K., Balleza, E., Kiviet, D. J., Hauschild, R., et al. (2017). Biased partitioning of the multidrug efflux pump AcrAB-TolC underlies long-lived phenotypic heterogeneity. Science 356, 311–315. doi: 10.1126/science.aaf4762
Chang, C., Garcia-Alcala, M., Saiz, L., Vilar, J. M. G., and Cluzel, P. (2022). Robustness of DNA looping across multiple cell divisions in individual bacteria. PNAS 119:e2200061119. doi: 10.1073/pnas.2200061119
Cluzel, P., Surette, M., and Leibler, S. (2000). An ultrasensitive bacterial motor revealed by monitoring signaling proteins in single cells. Science 287, 1652–1655. doi: 10.1126/science.287.5458.1652
Cohen, S. P., Levy, S. B., Foulds, J., and Rosner, J. L. (1993). Salicylate induction of antibiotic resistance in escherichia coli: activation of the mar operon and a mar-independent pathway. J. Bacteriol. 175, 7856–7862. doi: 10.1128/jb.175.24.7856-7862.1993
Elowitz, M. B., and Leibler, S. (2000). A synthetic oscillatory network of transcriptional regulators. Nature 403, 335–338. doi: 10.1038/35002125
George, A. M., and Levy, S. B. (1983). Amplifiable resistance to tetracycline, chloramphenicol, and other antibiotics in escherichia coli: involvement of a non-plasmid-determined efflux of tetracycline. J. Bacteriol. 155, 531–540. doi: 10.1128/jb.155.2.531-540.1983
Guet, C. C., Bruneaux, L., Min, T. L., Siegal-Gaskins, D., Figueroa, I., Emonet, T., et al. (2008). Minimally invasive determination of mrna concentration in single living bacteria. Nucleic Acids Res. 36:e73. doi: 10.1093/nar/gkn329
Guet, C. C., Elowitz, M. B., Hsing, W. H., and Leibler, S. (2002). Combinatorial synthesis of genetic networks. Science 296, 1466–1470. doi: 10.1126/science.1067407
Kim, J. M., Garcia-Alcala, M., Balleza, E., and Cluzel, P. (2020). Stochastic transcriptional pulses orchestrate flagellar biosynthesis in Escherichia coli. Sci. Adv. 6. doi: 10.1126/sciadv.aax0947
Le, T. T., Emonet, T., Harlepp, S., Guet, C. C., and Cluzel, P. (2006). Dynamical determinants of drug-inducible gene expression in a single bacterium. Biophys. J. 90, 3315–3321. doi: 10.1529/biophysj.105.073353
Le, T. T., Harlepp, S., Guet, C. C., Dittmar, K., Emonet, T., Pan, T., et al. (2005). Real-time RNA profiling within a single bacterium. PNAS 102, 9160–9164. doi: 10.1073/pnas.0503311102
Lewis, K. (2007). Persister cells, dormancy and infectious disease. Nat. Rev. Microbiol. 5, 48–56. doi: 10.1038/nrmicro1557
Li, X. Z., and Nikaido, H. (2004). Efflux-mediated drug resistance in bacteria. Drugs 64, 159–204. doi: 10.2165/00003495-200464020-00004
Locke, J. C. W., and Elowitz, M. B. (2009). Using movies to analyse gene circuit dynamics in single cells. Nat. Rev. Microbiol. 7, 383–392. doi: 10.1038/nrmicro2056
Lutz, R., and Bujard, H. (1997). Independent and tight regulation of transcriptional units in escherichia coli via the lacR/o, the tetR/o and arac/i-1-i-2 regulatory elements. Nucleic Acids Res. 25, 1203–1210. doi: 10.1093/nar/25.6.1203
Martin, R. G., Bartlett, E. S., Rosner, J. L., and Wall, M. E. (2008). Activation of the escherichia coli mara/soxs/rob regulon in response to transcriptional activator concentration. J. Mol. Biol. 380, 278–284. doi: 10.1016/j.jmb.2008.05.015
Martin, R. G., Jair, K. W., Wolf, R. E., and Rosner, J. L. (1996). Autoactivation of the marrab multiple antibiotic resistance operon by the mara transcriptional activator in escherichia coli. J. Bacteriol. 178, 2216–2223. doi: 10.1128/jb.178.8.2216-2223.1996
Martin, R. G., and Rosner, J. L. (1995). Binding of purified multiple antibiotic-resistance repressor protein (Marr) to mar operator sequences. PNAS 92, 5456–5460. doi: 10.1073/pnas.92.12.5456
Martin, R. G., and Rosner, J. L. (2004). Transcriptional and translational regulation of the MarRAB multiple antibiotic resistance operon in escherichia coli. Mol. Microbiol. 53, 183–191. doi: 10.1111/j.1365-2958.2004.04080.x
Meouche, I. E., and Dunlop, M. J. (2018). Heterogeneity in efflux pumpexpression predisposesantibiotic-resistant cells to mutation. Science. 362, 686–690. doi: 10.1126/science.aar7981
Nagai, T., Ibata, K., Park, E. S., Kubota, M., Mikoshiba, K., and Miyawaki, A. (2002). A variant of yellow fluorescent protein with fast and efficient maturation for cell-biological applications. Nat. Biotechnol. 20, 87–90. doi: 10.1038/nbt0102-87
Novick, A., and Weiner, M. (1957). Enzyme induction as an all-or-none phenomenon. Proc. Natl. Acad. Sci. U. S. A. 43, 553–566. doi: 10.1073/pnas.43.7.553
Rosner, J. L. (1985). Nonheritable resistance to chloramphenicol and other antibiotics induced by salicylates and other chemotactic repellents in Escherichia coli K-12. PNAS 82, 8771–8774. doi: 10.1073/pnas.82.24.8771
Siegele, D. A., and Hu, J. C. (1997). Gene expression from plasmids containing the araBAD promoter at subsaturating inducer concentrations represents mixed populations. PNAS 94, 8168–8172. doi: 10.1073/pnas.94.15.8168
Stauffer, D., and Aharony, A. (1994). Introduction to percolation theory. Taylor & Francis, London: CRC Press.
Taniguchi, Y., Choi, P. J., Li, G. W., Chen, H., Babu, M., Hearn, J., et al. (2010). Coli proteome and transcriptome with single-molecule sensitivity in single cells. Science 329, 533–538. doi: 10.1126/science.1188308
Thomas, R., and Richelle, J. (1988). Positive feedback loops and multistationarity. Discret. Appl. Math. 19, 381–396. doi: 10.1016/0166-218X(88)90026-1
Vilar, J. M. G., Guet, C. C., and Leibler, S. (2003). Modeling network dynamics: the lac operon, a case study. J. Cell Biol. 161, 471–476. doi: 10.1083/jcb.200301125
Wen, X., Langevin, A. M., and Dunlop, M. J. (2018). Antibiotic export by efflux pumps affects growth of neighboring bacteria. Sci Rep. 8:15120. doi: 10.1038/s41598-018-33275-4
Keywords: single cell, gene expression, bacteria, heterogeneity, non genetic memory
Citation: Guet CC, Bruneaux L, Oikonomou P, Aldana M and Cluzel P (2023) Monitoring lineages of growing and dividing bacteria reveals an inducible memory of mar operon expression. Front. Microbiol. 14:1049255. doi: 10.3389/fmicb.2023.1049255
Edited by:
Hanna Salman, University of Pittsburgh, United StatesReviewed by:
María Antonia Sánchez-Romero, Sevilla University, SpainSaurabh Mishra, Cornell University, United States
Copyright © 2023 Guet, Bruneaux, Oikonomou, Aldana and Cluzel. This is an open-access article distributed under the terms of the Creative Commons Attribution License (CC BY). The use, distribution or reproduction in other forums is permitted, provided the original author(s) and the copyright owner(s) are credited and that the original publication in this journal is cited, in accordance with accepted academic practice. No use, distribution or reproduction is permitted which does not comply with these terms.
*Correspondence: Calin Guet, calin@ist.ac.at
†These authors have contributed equally to this work